Article
DOI:
https://doi.org/10.18845/te.v16i2.6186
What drives project management success in developing countries? The case of Ghana
¿Qué favorece el éxito en la gestión de proyectos en los países en desarrollo? El caso de Ghana
TEC Empresarial, Vol. 16, n°. 2, (May - August, 2022), Pag. 55 - 78, ISSN: 1659-3359
AUTHORS
Armstrong Amoah*
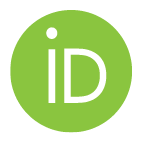
Faculty of IT Business, Ghana Communication Technology University, Ghana.
aamoah@gctu.edu.gh.
Jasmina Berbegal-Mirabent
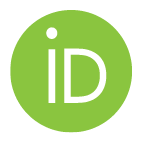
Department of Management, Universitat Politècnica de Catalunya, Spain.
jasmina.berbegal@upc.edu.
Frederic Marimon
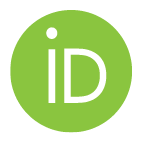
Department of Economy and Business Organisation, Universitat Internacional de Catalunya, Spain.
fmarimon@uic.es.
Corresponding Author: Armstrong Amoah
ABSTRACT
Abstract
Many projects in developing countries (DCs) have been recognised as crucial forces for poverty reduction and making people’s lives easier. Despite their relevance, projects in these countries typically fail or are abandoned before completion. Recent calls suggest that projects in DCs are multifaceted, interdependent and of a complex nature; consequently, they are influenced by various factors that are specific to these territories, requiring new research efforts that delve deeper into the particularities of this specific setting. Literature on project management success (PMS) in DCs is very limited, and the existing attempts either rely on PMS factors for developed countries and/or use conventional variable-oriented analyses that are not transferrable to the context of DCs. Aiming to shed new light on the factors that shape PMS in DCs, this study examines both the configurational and the independent influence of three factors that have been emphasised in project management literature as determinants of PMS. To achieve this objective, a survey was conducted among 215 project management practitioners in Ghana. First, we validate the factors’ structure obtained from literature. Next, we examine the configurational influence of these factors on PMS in DCs, complementing the findings with robustness checks. Our results support the existence of distinct pathways, suggesting that there is no unique recipe, but different strategies that can be followed. The study concludes with a series of recommendations that are expected to help engineers and project managers in DCs understand and deal with project management challenges.
Keywords: Organisational capacity, organisational structure, leadership, project management success, qualitative comparative analysis, structural equation modelling.
Resumen
Los proyectos de construcción en los países en desarrollo (PED) son una fuerza crucial para reducir la pobreza y facilitar la vida de las personas. A pesar de su relevancia, dichos proyectos suelen fracasar o abandonarse antes de su finalización. Recientes discusiones sugieren que los proyectos en los PED son multifacéticos, interdependientes y de naturaleza compleja, estando influenciados por factores específicos de estos territorios, lo que requiere de nuevos estudios que profundicen en las particularidades de este contexto. Esta cuestión se ha tratado poco en la literatura, y los intentos existentes se fundamentan en los factores utilizados para caracterizar el éxito en los países desarrollados y/o utilizan análisis convencionales que no son transferibles al contexto de los PED. Con el objetivo de determinar qué factores conducen al éxito en la gestión de proyectos en los PED, este estudio examina la influencia configuracional y la independencia de tres factores que tradicionalmente se consideran determinantes del éxito. La aplicación empírica analiza 215 respuestas de gestores de proyectos de Ghana. Primero, se validan los elementos que constituyen los factores clave que intervienen en la gestión de proyectos. A continuación, se examina cómo dichos factores se combinan entre ellos para dar lugar a configuraciones que resultan en proyectos exitosos en un contexto de PED. Los resultados apoyan la existencia de distintas configuraciones, sugiriendo que no hay una propuesta única sino distintas estrategias posibles a seguir. El estudio concluye con recomendaciones para comprender y enfrentar mejor los desafíos de la gestión de proyectos.
Palabras clave: Capacidad organizacional, estructura organizacional, liderazgo, éxito en la gestión de proyectos, análisis comparativo cualitativo, modelado de ecuaciones estructurales.
Introduction
1. Introduction
A project can be defined as an endeavour in which human, material and financial resources are organised in a novel way to undertake a unique scope of work, comprising a given set of specifications within cost and time constraints, seeking to achieve beneficial change defined by quantitative and qualitative objectives (Project Management Institute, 2017). Projects usually involve unique and transient efforts, and are undertaken to achieve planned objectives which can be defined in terms of outputs, outcomes or benefits (International Project Management Association, 2006). The achievement of the abovementioned planned objectives depends greatly on how the project is managed (Alias et al., 2014).
Within this context, project management (PM) can be understood as the planning, organisation, monitoring and control of all aspects of a project, motivating all project stakeholders to achieve the project goals in a safe manner and within the agreed schedule, budget and performance criteria (Radujkovića & Sjekavicab, 2017). This definition suggests that in PM there is a strong focus on performance and whether success is accomplished. Therefore, project management success (PMS) refers to the level of efficiency the project achieves to reach its objectives (Siles, 2021). A successfully managed project is one that is completed within time, the cost of the project being within budget and meeting the initial planned performance (Tesfaye et al., 2017).
PM has become a very popular and effective tool that is not only used by corporations to achieve their business objectives, but also by public administrations to boost their developmental agenda (Ofori, 2013). Certainly, PM methods have been extensively used by many public and private entities to solve their problems, manage scarce resources and achieve important objectives (Essilfie-Baiden, 2019). The wide variety of applications of its practice reinforces the need to train professionals in this area so that they can lead teams and improve the project’s chances of success. This is particularly relevant in the case of developing countries (DCs). According to Ofori-Kuragu et al. (2016), the policies introduced by governments are usually converted to projects and are recognised as the channels through which governmental initiatives can be implemented. The impact of government interventions is thus dependant on how these projects are managed. Given the limited resources of DCs, efficient PM in such countries is of paramount importance.
Unfortunately, the rate of PM failure in DCs and its consequences, such as cost overrun, is a fact, and has become a matter of concern. Recent works in several DCs have evidenced that a high percentage of projects incur cost overruns and delays (e.g. Asiedu & Adaku, 2019; Damoah & Akwei, 2017; Ullah et al., 2018). In the same vein, Kamaruddeen (2020) mentioned that only 16 per cent of 8,000 construction projects in Nigeria were completed within budget, on time and at the required level of quality. Ika (2012) stated that many obstacles, described as notorious and critical implementation problems, impede PMS. While some are easy to deal with, others are almost uncontrollable. PM in many DCs faces problems related to execution and governance, limiting their successful completion, and of those that come to an end, the vast majority are not completed as initially projected (Amoah et al., 2021). Likewise, projects in DCs, and particularly those that respond to governmental strategies, typically have to deal with non-technical problems such as a large number of participants, poor procurement systems, bureaucratic processes, lack of skills and resources, and complex procedures (Williams, 2017). The result is a number of incomplete, misleading or delayed projects (Fong et al., 2006).
The reason that some projects that are successfully implemented in developed countries fail when replicated in DCs is not because the projects are deficient or are not conducive for DCs, but rather because they are not properly assessed and managed in the peculiar and complex context of these countries (Yanwen, 2012). PM challenges in DCs are usually addressed as if they were happening in more favourable territories. DCs do not have the same socio-economic environment as developed countries; therefore, project managers need specific skills and competences to manage the complexity of projects successfully in such a challenging context (Amoah & Marimon, 2021).
Previous studies have signalled organisational capacity, organisational structure and leadership as indispensable factors that significantly influence PMS (Aniagyei, 2011; Blaskovics, 2014; Hyväri, 2006); however, these studies have neglected the specificities of DCs, being conducted mainly in wealthy economies. In addition, most of these works used conventional variable-oriented analyses (VOA) to investigate the independent effects of each of these factors on PMS. The use of VOA offers a narrow approach in the finality of whether each variable is valuable or not in predicting a specific outcome, hindering any interpretative approach explaining causation with a set of variables moving jointly. VOA assumes symmetrical relationships between variables, when the reality is far more complex (Lee, 2008). Furthermore, most VOAs build on linear additive models that aim at measuring the unique contribution of each independent variable to a dependent variable and do not report three-way or four-way interaction, and if they do it, they do so with great difficulty explaining such terms (Woodside, 2010).
While VOAs have brought valuable insights for project initiators to recognise critical factors that require attention for effective PM, they have underestimated the fact that projects in DCs are multifaceted, interdependent and complex in nature (Ackah, 2020; Murch, 2001). This implies that studying the effects of critical success factors independently is not sufficient. The use of qualitative comparative analysis (QCA) is therefore recommended. QCA studies cases as configurations of causes and conditions, thus increasing the possibility of diverse interpretations. It allows a higher degree of flexibility in terms of examining causal complexities under the rubric of multiple conjunctural causation, and accurately expresses the various routes to a given outcome. This characteristic is particularly attractive for the purpose of our study, as the asymmetry in the relationships between the factors (organisational capacity, organisational structure and leadership) and the outcome (PMS) deserves further investigation, particularly in DCs (Ofori, 2013; Yanwen, 2012). By using QCA we do not impose one unique formula, but instead allow project-oriented organisations to combine their limited resources and capabilities freely to find their path to PMS.
Based on the previous arguments, this study seeks (1) to validate three major factors (namely, organisational capacity, organisational structure and leadership) that, according to the literature, influence PMS in the context of DCs; (2) to employ alternative analytical techniques to reveal which configuration(s) of these factors influence PMS in DCs; and, based on these, (3) to draw managerial and policy implications with a special focus on DCs.
The remainder of the article is structured as follows. After this introduction, we dive deeper into the theoretical foundations that support this research and review the three main factors included in our framework. Next, we describe the data collection process and the methodology to test our model empirically. Afterwards, the results are reported, followed by the discussion and the implications of the findings. The paper ends with the concluding remarks, limitations and suggestions for future research.
2. Theoretical underpinnings
Like many other disciplines, PM is a dynamic field in continuous evolution; therefore, what constituted PM success some years ago might no longer apply. Nevertheless, we can come up with a broad definition of PMS that puts the emphasis on the convergence of the ability of a process to meet the technical goals of a given project whilst not deviating from the constraints of scope, time and cost, and the usefulness of the project as perceived by beneficiaries, sponsors and the project team (Alias et al., 2014; Milosevic & Patanakul, 2005).
This broad definition opens the door to multiple subtleties in the interpretation. Not surprisingly, different authors have come up with various PMS factors, resulting in a lack of consensus among researchers on the criteria for judging PMS (Fortune & White, 2006). Diverse contexts and situations contribute to enlarge the list of critical success factors for PMS (Frefer et al., 2018). Nevertheless, the existing literature tends to use the traditional “iron triangle”—cost, quality and schedule—as the main criteria for measuring PMS (Walker & Shen, 2002); yet these factors can be operationalised in a number of ways. More recently, the literature has emphasised the skills necessary to manage a project effectively and combined the three aforementioned dimensions with competences and abilities such as good planning, clear responsibility and accountability, effective leadership, governance and communication, among others (Amoah & Marimon, 2021; Ahmed & Mohamad, 2016).
For the purpose of this study, we draw upon the model proposed in the works by Aniagyei (2011), Blaskovics (2014) and Hyväri (2006), whose settings included both developed countries and DCs, considering three main factors: organisational capacity, organisational structure and leadership. Note that these factors have been widely examined in developed countries and practical examples are widely documented, yet their use in DCs is still limited. Therefore, in the paragraphs that follow, we elaborate on how they can be operationalised taking into account the particularities of DCs.
2.1 Organisational capacity
Organisational capacity refers to the ability of an organisation to use its skills and resources effectively to achieve its objectives and satisfy its stakeholders’ requirements (Bjorvatn & Wald, 2018). These skills and resources include staffing, infrastructure, technology, financial resources, strategic leadership, process management, networks and linkages with other organisations and groups. Consequently, the capacity of an organisation involves all the components it takes for an organisation to achieve its mission, from physical resources to people and ideas. It involves an organisation’s ability to execute tasks, or the enabling factors that allow an organisation to execute its duties and achieve its objectives effectively (Cox et al., 2018). Simplifying this definition, the World Bank (2019) describes organisational capacity as the ability of an institution to use its skills, assets and resources to attain its objectives.
Recent studies have emphasised the critical role organisational capacity plays in achieving PMS. Bjorvatn and Wald (2018) and Hanisch et al. (2009) argued that successful PM is dependent on an organisation’s human, financial and technical capacities. Consequently, organisational capacity has been found to have a direct effect on the quality, time spent and overall success of a single project (Rankonyana, 2015). Therefore, embarking on effective monitoring and evaluation of an organisation’s capacity development is of critical importance to ensure that capacity development initiatives actually lead to PMS (Cox et al., 2018).
In their attempt to operationalise organisational capacity, Muriithi et al. (2016) used determinants such as human resources (e.g. personnel, roles and responsibilities); strategic leadership (e.g. vision and mission, mandate, organisational structure, organisational goals and objectives, motivation, incentives and appraisal procedures); financial resources (e.g. budgetary allocations); infrastructure (e.g. technology and facilities); processes management (e.g. processes and knowledge sharing and building); and the external environmental (e.g. legal and administrative environments, political will, policies, and networks and partnerships with other stakeholders). Using a more summarised approach, Connolly and Lukas (2002) mentioned the determinants of organisational capacity which they observed to be critical for PMS: mission, vision and strategy, governance and strategic leadership, finance, internal operations and management, and program delivery. Other determinants commonly used in the PM literature include leadership capacity, operational capacity, management capacity, adaptive capacity (Engle, 2011), structure or governance, skills, human capital and accountability (Cox et al., 2018).
2.2 Organisational structure
Organisational structure is the prescribed system of work and communication relationships that directs, integrates and motivates employees to work together towards the achievement of the organisation’s objectives (Ochieng, 2016). The structure of an organisation involves the methods used to divide, categorise and integrate tasks (Sablynski, 2012), along with the framework used to supervise the organisation’s operations (Ubani, 2012).
In the field of PM, the structure of an organisation has been found to have a significant effect on PMS. Studies show that the structure of a project-oriented organisation largely affects the way the project team interacts and works, which subsequently impacts the organisation’s ability to manage its projects successfully (Wallace, 2007). Consequently, organisations have been advised to be cautious about their structure since it influences their productivity, efficiency and effectiveness in terms of PM (Ubani, 2012). Also, it affects the authority of the project manager to make decisions regarding information dissemination and the integration of the construction aspect of the project (Ekundayo et al., 2013), and permits the arrangement of tasks with respect to different functional groups that work with each other in PM (Inuwa & Kunya, 2015).
Early conceptualisations of the construct of organisational structure resorted to the use of organisational complexity, formalisation, centralisation, decentralisation of authority, technological demands, organisational growth, environmental turbulence and size, and business strategy (Mukalula, 1996). Pennings (2002) divided the determinants in two broad categories: centralisation (which includes employees’ involvement in decision-making, classification of authority and departmental participation in decision-making) and formalisation (which includes job codification, job specificity, strictness, rule observation and written communication), while Robbins and Bamwell (2006) distinguished five dimensions: strategy, organisational size, technology, environment and power control. Also, the work of Ubani (2012) in the context of civil engineering projects is remarkable, where he used span of control, level of flexibility, supervisors’ competence and level of lean staffing, number of divisions/departments, levels of power/authority and communication flow, accountability and quality-oriented indices, and level of simplicity.
2.3 Leadership
Leadership can be defined as an influencing process between superiors and subordinates towards the achievement of organisational objectives (Lussier & Achua, 2013). It involves the chain of command in an organisation from which authority flows. People in leadership are expected to perform certain roles over other employees and are expected to assign tasks and responsibilities to their subordinates (Ochieng, 2016). To accomplish this task, leaders should have the ability to make decisions, have energy, a sense of duty, confidence and pride in command, and be able to influence followers to engage in achieving organisational objectives, using appropriate motivational techniques of influence based on power and formal or informal authority (Islam et al., 2017).
The impact of leadership on PMS has been recognised by Burke et al. (2006), who stated that without dynamic leadership, the project team responsible for the delivery of the organisation’s project objectives would be like a rudderless boat. Similarly, Turner (2006) argued that in the context of PM, leadership ensures compliance with procedures, the establishment of appropriate relationships, and communication of the values of the organisation, resulting in PMS. According to Kerzner (2013), there are four vital components when exercising good PM leadership methodologies, these being effective communication, effective co-operation, effective teamwork and trust, all having a significant impact on PMS. Based on the above arguments, since leadership plays an important role in achieving PMS, the project manager is expected to pay special attention to his management and leadership roles (Pandya, 2014).
Measurement of the attributes of leadership in PM differs from study to study, although each approach tries to highlight the areas in which a leader must excel. For instance, Meredith and Mantel (2011) identified the quality of technical skills, political awareness, the kind of orientation towards achieving stated objectives, and level of self-esteem. Larson and Gray (2014) focused on being a systems thinker and skilful politician with personal integrity, pro-activeness, emotional intelligence, a general business perspective, time management and optimism as determinants of leadership. Dulewicz and Higgs (2004) developed three categories of determinants of leadership: managerial (resource management, engaging, communicating, empowering development and achievement); intellectual (detailed analysis and judgement, vision, and creativity and strategy); and emotional (self-recognition, emotional resilience, intuitiveness, sensitivity influence, motivation and consciousness). A more recent characterisation of leadership is that proposed by Sienkiewicz-Malyjurek (2016) which includes innovativeness, coherence, inventiveness, conciseness, confidence, consistency, open-mindedness and competence.
2.4 The Ghanaian context
Ghana is the country chosen for the empirical application. Like many DCs, Ghana faces several setbacks in the management of its projects (Darko & Löwe, 2016). Studies on this country report a lot of incomplete projects that have not yielded any benefits to the citizenry (Kissi et al., 2015). Projects, which are a significant part of the economy of Ghana, have been highlighted as a priority in most of the country’s budget statements (Budget of the Republic of Ghana, 2019; Damoah & Akwei, 2017). Nevertheless, in most cases, the outcome obtained from the implementation of these projects has not met the goals set at the beginning in terms of time, cost and quality (Ofori-Kuragu et al. 2016). What is more, as Damoah and Kumi (2018) observed, many projects in Ghana not only fail to achieve their anticipated objectives but are completely abandoned half way through. Researchers have often attributed these PM failures to a lack of understanding of the complex, multi-faceted and interdependent nature of PM in Ghana (Ofori-Kuragu et al., 2016). It is worth noting that the aforementioned problems are not specific to Ghana but are commonly shared by many DCs (Owusu-Ansah & Louw, 2019). Thus, the selection of this country for the study is deemed appropriate.
Methods
3. Methodology
3.1 Sample Design and Data Collection
In order to obtain data on the role of organisational capacity, organisational structure and leadership in achieving PMS in DCs, a survey targeted certified PM practitioners in Ghana, of who, according to the Project Management Institute of this country, there were 442. The profile of the survey participants includes government officials, heads of public and private institutions, civil servants who give project contracts, contractors and managers, managers of non-governmental organisations, and employees of project-oriented organisations. Using Sekaran’s (2009) probability sampling table, 220 PM practitioners were selected for the survey using the purposive sampling technique.
The survey was self-administered by the executives of the Project Management Institute in Ghana for onward distribution to its members in December 2019. This was done after a letter of request explaining the purpose of the study and requesting their agreement to participate in the survey. In an endeavour to acquire the minimum sample size, the authors distributed the survey to a total of 220 respondents, of whom 219 returned responses (representing a response rate of 95.2%) after completion during the period December 2019 to February 2020. After discarding surveys containing incomplete information, 215 responses were finally considered as valid. The main demographic characteristics of the respondents are summarised in Table 1.
3.2 Measures
The survey was designed using the English language and consisted of two parts. Part one, including five items, requested that respondents provide demographic information. Part two contained a series of statements grouped in three factors (i.e. organisational capacity, organisational structure and leadership) that were operationalised through 16 items. Six additional items were included to capture information about the outcome of interest (PMS), making a total of 22 items.
Number |
% |
|
Gender |
||
Male |
171 |
79.50 |
Female |
44 |
20.58 |
Total |
215 |
100.00 |
Age |
||
18-20 |
4 |
1.90 |
21-31 |
73 |
34.00 |
31-40 |
91 |
42.30 |
41-50 |
34 |
15.80 |
> 50 |
13 |
6.00 |
Total |
215 |
100.00 |
Education |
||
Certificate |
9 |
4.20 |
Diploma |
29 |
13.50 |
Bachelors |
109 |
50.70 |
Masters |
65 |
30.20 |
Doctorate |
3 |
1.40 |
Total |
215 |
100.00 |
Work Experience (Years) |
||
Below 1 |
9 |
4.20 |
1 – 5 |
83 |
38.60 |
6 – 10 |
66 |
30.70 |
Above 10 |
57 |
26.50 |
Total |
215 |
100.00 |
PM Certification |
||
PMP |
130 |
60.50 |
CAPM |
68 |
31.60 |
PMI-RMP |
7 |
3.30 |
PMI-PBA |
3 |
1.40 |
OTHER |
7 |
3.30 |
Total |
215 |
100.00 |
A five-point Likert scale (1 denoting “strongly disagree”, 5 denoting “strongly agree”) was used when asking respondents to express their level of agreement with each of the 22 statements included in the survey. The scales to measure the three main constructs of interest were adapted and modified from those proposed in the studies by Aniagyei (2011), Blaskovics (2014) and Hyväri (2006). The selection of these scales was as a result of their fit with the objectives of this study. Furthermore, the scales have been already validated and tested for reliability, and thus can serve as a baseline. Table 2 contains the detailed list of items.
Organisational structure |
Organisational structure-systems and structure |
OSss1 |
Formalisation |
OSss2a |
Explicit rules, regulations, policies and procedures govern organisational activities |
||
OSss3 |
Communication flow |
||
Organisational structure-span of control |
OSspc4 |
Span of control |
|
OSspc5 |
Bureaucracy |
||
OSspc6 |
Centralisation |
||
Leadership |
LEAD1 |
Leadership skills and behaviours |
|
LEAD2 |
Leaders technical knowledge |
||
LEAD3 |
Open to creativity, innovation and new ideas |
||
LEAD4 |
Leaders ability to connect with others in a positive way |
||
LEAD5 |
Personal Integrity |
||
Organisational capacity |
OC1 |
Organisational governance |
|
OC2 |
Availability of enough competent human capital |
||
OC3 |
Enough technical capacity to carry out complex projects |
||
OC4 |
Well laid down legal structures |
||
OC5 |
Availability of adequate IT infrastructure to support complex PM activities |
||
Project management success |
PMS1 |
Project schedule management |
|
PMS2 |
Project cost management |
||
PMS3 |
Project risk management |
||
PMS4 |
Project quality management |
||
PMS5 |
Costumers expectations management |
||
PMS6 |
Project team management |
3.3. Method
To corroborate the suitability of the data for factor analysis, the Kaiser–Meyer–Olkin (KMO) test and the Bartlett’s test of sphericity (Mikkelsen, 2019) were conducted (see Table 3). Results provided a KMO test value of 0.799, exceeding the recommended threshold value of 0.60, thus indicating good sampling adequacy and that data were suitable for factor analysis. The Bartlett’s test of sphericity was also positive and significant at 0.05 (i.e. χ² = 1424.042, df = 120, p = 0.000 < 0.05), confirming a linear relationship between the variables (Meyer & Collier, 2001; Pallant, 2010). Both tests were performed using SPSS for Windows v25.
Next, to validate the factor structure of the measurement variables, an exploratory factor analysis was run using principal components analysis and varimax rotation. To ensure the overall consistency of our measures, the reliability analysis of the factors obtained was confirmed using Cronbach’s alpha and composite reliability. The discriminant validity analysis was conducted using the standardised covariances between the latent factors and examining whether the inter-factor correlations were lower than the square root of the average variance extracted (AVE).
Once the factors were established, in the next step we examined how they combine in order to facilitate PMS. Identification of the combination(s) of factor(s) yielding a certain outcome calls for the use of configurational approaches. Qualitative comparative analysis (QCA) is the preferred method for addressing this type of research question (Schneider & Wagemann, 2012). QCA is a hybrid method that combines the advantages of both qualitative analysis (case-oriented research) and quantitative analysis (variables-oriented research). QCA helps to explore equifinality—that is, how multiple causal paths underline a certain phenomenon (Salam et al., 2017). One of the distinctive features of this method is that it uses Boolean logic instead of the traditional correlation methods to set causal conditions strongly related to a particular outcome (Ragin, 2008). This technique relies on the analysis of sufficient and necessary conditions to produce an outcome, and has strengths for small to medium-level case studies. A condition is deemed necessary if it is present in all instances of the outcome, while a condition will be considered sufficient if a particular outcome emerges whenever the condition is present (Schneider & Wagemann, 2012).
KMO and Bartlett’s Test |
||
Kaiser-Meyer-Olkin Measure of Sampling Adequacy. |
0.799 |
|
Bartlett’s Test of Sphericity |
Approx. Chi-Square |
1424.042 |
df |
120 |
|
Sig. |
0.000 |
QCA is particularly relevant to the context of this study because the factors explaining PMS are multifaceted and complex in nature. This is due to the inherent complexity a project entails and the presence of distinct participants in the PM process. Thus, studying the effects of these factors independently will not be enough to provide a clear understanding of the problem (Ofori, 2013; Yanwen, 2012). Instead of assessing the individual effect of each condition on the outcome, we evaluate cases as configurations of conditions (Ragin, 2008). Another reason for the use of QCA is that this technique is better suited for conducting an assessment in a specific context, showing how multiple causal recipes relate to a particular outcome and help answer certain questions that could not be addressed via conventional variables-oriented analyses (Ragin, 2008).
QCA has two main variants: crisp-set qualitative comparative analysis (csQCA) and fuzzy-set qualitative comparative analysis (fsQCA). For the purpose of this study, we used fsQCA, with the aid of the fsQCA 3.0 software. The greatest difference between csQCA and fsQCA is that the former utilises the traditional aggregation concept using 0 (out of the set) and 1 (inside the set), while fsQCA accounts for varying degrees of membership between 0 (fully out of the set) and 1 (fully in), therefore minimising the loss of information. Because of the nuanced nature of the conditions influencing our outcome, fsQCA was selected over csQCA (Choi, 2009).
Results
4. Results
4.1 Scale validation
To help reduce and group similar items into appropriate dimensions, factor analysis was conducted using principal components analysis and varimax rotation. The 16 items included in the survey were used at this step. To retain an item, we imposed the following criteria: the item (a) loaded at 0.6 or more on a factor; (b) did not load at greater than 0.5 on two factors; (c) had an item-to-total correlation of greater than 0.5; and (d) had an eigenvalue greater than 1 (Ladhari, 2012). In total, four factors emerged from our analyses with eigenvalues greater than 1 (Kaiser, 1960), explaining 65.97 per cent of the variance in the sample, as shown in Table 4.
EFA (16 ITEMS) |
||||
2-LEAD |
3-OSss |
1-OC |
4-OSspc |
|
LEAD2 |
0.896 |
0.029 |
-0.027 |
-0.012 |
LEAD3 |
0.846 |
-0.068 |
-0.120 |
0.088 |
LEAD4 |
0.823 |
-0.020 |
0.042 |
0.141 |
LEAD1 |
0.752 |
0.156 |
-0.125 |
-0.043 |
OSss1 |
-0.070 |
0.796 |
-0.034 |
0.214 |
OSss3 |
-0.039 |
0.792 |
-0.025 |
0.227 |
OC5 |
0.097 |
0.757 |
-0.028 |
0.243 |
OSss2 |
0.148 |
0.705 |
0.116 |
0.172 |
LEAD5 |
-0.032 |
0.481 |
-0.061 |
0.435 |
OC2 |
0.036 |
0.112 |
0.834 |
-0.100 |
OC3 |
0.078 |
0.177 |
0.796 |
-0.124 |
OC4 |
-0.152 |
-0.182 |
0.792 |
0.206 |
OC1 |
-0.275 |
-0.158 |
0.791 |
0.097 |
OSspc4 |
0.128 |
0.230 |
0.001 |
0.735 |
OSspc5 |
0.061 |
0.260 |
0.021 |
0.729 |
OSspc6 |
-0.010 |
0.331 |
0.034 |
0.717 |
% of Variance |
18.302 |
18.274 |
16.469 |
12.922 |
New labels were proposed after the groupings from the factor analysis, given the relatively few overlaps with the original dimensions. Even though the model for this study is made up of three latent factors, four factors emerged from the factor analysis and were therefore used in the next steps. Specifically, organisational structure split into two sub-factors, which have been labelled organisational structure (systems and structures) (OSss) and organisational structure (span of control) (OSspc). The two remaining factors are organisational capacity (OC) and leadership (LEAD).
To examine the uni-dimensionality of the newly obtained factors, four new independent factor analyses using an orthogonal rotation method (varimax) were conducted, each of them containing the items identified in the previous step (the shaded items in Table 4). The four factors extracted only one factor each, validating our approach. Table 5 provides the statistics for reliability and convergent validity for the four factors, as well as the loading of those items that significantly contribute to explain each factor (loadings > 0.66). In all instances the Cronbach’s alpha and the composite reliability exceeded the threshold value of 0.7 for internal consistency (Shemwell et al., 2015). Also, the AVE (dos Santos & Cirillo, 2021) of each factor was greater than 0.5, which is the benchmark of the required threshold (Fornell & Larcker, 1981), suggesting a good fit.
1 |
2 |
3 |
4 |
|||||
OC |
LEAD |
OSss |
OSspc |
|||||
OC1 |
0.825 |
LEAD1 |
0.762 |
OSss1 |
0.827 |
OSspc4 |
0.809 |
|
OC2 |
0.815 |
LEAD2 |
0.900 |
OSss2 |
0.752 |
OSspc5 |
0.791 |
|
OC3 |
0.776 |
LEAD3 |
0.856 |
OSss3 |
0.814 |
OSspc6 |
0.811 |
|
OC4 |
0.818 |
LEAD4 |
0.830 |
OC5 |
0.830 |
|||
Alpha Cronbach |
0.823 |
0.846 |
0.818 |
0.754 |
||||
Range of Cronbach’s alpha if an item is deleted |
0.768 - 0.796 |
0.754 - 0.858 |
0.754 - 0.803 |
0.625 - 0.752 |
||||
Range of total corrected scale and correlations |
0.606 - 0.666 |
0.605 - 0.796 |
0.575 - 0.675 |
0.519 - 0.627 |
||||
Composite Reliability |
0.883 |
0.904 |
0.881 |
0.846 |
||||
Average Variance Extracted (AVE) |
0.654 |
0.703 |
0.650 |
0.646 |
*** All loads significant at p-value = 0.01
With the exception of LEAD, the Cronbach’s alpha values did not improve when one of the items was deleted from the scales for each dimension, and the correlations between each item and the total corrected scales were all above 0.5. In the specific case of LEAD, we decided not to remove any item because the new Cronbach’s alpha when doing so was not significantly different from the initial one. All the items were shown to have significant loads (t > 2.58); thus convergent validity was confirmed for all the factors (Malhotra, 1999).
To establish the extent to which factors are distinct and uncorrelated, and to confirm whether dissimilar measures are in fact unrelated, discriminant validity analysis was carried out. Table 6 shows the results for the analysis of the discriminant validity, which was conducted using linear correlations of standardised covariances among latent factors by investigating whether the inter-factor correlations were below the square root of the AVE (Fornell & Larcker, 1981). Note that the square root of each AVE was greater than the off-diagonal elements. Thus, discriminant validity was also verified (Hair et al., 2010).
Finally, we also controlled for potential common method bias using two approaches. Firstly, we used Harman’s single-factor test (Meyer et al., 1993). In this test, the first largest common factor accounted for 38.47 per cent of the total variance, revealing that no single construct accounted for more than half of all the variance (Podsakoff et al., 2003). Additionally, we examined the correlation matrix of the latent constructs as suggested by Pavlou et al. (2007). As shown in Table 6, there are no high correlations which could compromise the data. Therefore, we concluded that common method bias was not a major concern in this study.
4.2 Configurations that explain PMS in DCs
Next, a QCA was performed to investigate the causal paths that lead to PMS in DCs. The factors obtained after the factor analysis were used as the antecedent conditions, while PMS was used as the outcome. Data were transformed (i.e. calibrated, using the QCA nomenclature) into fuzzy-set terms, expressing the values of each observation according to their degree of membership from 0 (full non-membership) to 1 (full membership), 0.5 being the crossover point. Similar to Alrik and Dușa (2013) and Ragin (2008), observations falling in the 90th percentile represented the threshold of 0.95 (equivalent to full membership), while the 10th percentile was used as the 0.05 cut-off point (full non-membership). The median was used to proxy the cross-over point—i.e. observations with maximum ambiguity with respect to their membership in the set (see Table 7).
|
1 |
2 |
3 |
4 |
Organisational capacity |
0.809 |
|||
Leadership |
-0.161* |
0.839 |
||
Organisational structure-systems & structures |
-0.011 |
0.081 |
0.806 |
|
Organisational structure-span of control |
0.029 |
0.113 |
0.556** |
0.804 |
* Correlation significant at 0.05
** Correlation significant at 0.01
In the main diagonal are the square root of the AVE of each construct.
Condition |
Membership Threshold Values |
||
Full Non-membership |
Crossover point |
Full Membership |
|
Organisational capacity |
-1.55 |
0.16 |
1.13 |
Leadership |
-1.36 |
0.28 |
1.21 |
Organisational structure-systems & structures |
-1.44 |
0.12 |
1.04 |
Organisational structure-span of control |
-1.33 |
-0.03 |
1.16 |
Outcome |
|||
Project management success |
-1.06 |
-0.54 |
1.45 |
Testing for the necessity of the antecedent conditions in prediction of the desired outcome is one of the first steps in QCA (Meyer et al., 1993). Consistency scores greater than 0.9 indicate necessity (Ragin, 2008); however, as shown in Table 8, there is no antecedent condition that can predict the outcome alone. Thus these preliminary results seem to be in line with the initial intuition that a combination of the latent factors can provide more explanation for the outcome.
Next, the truth table was built. This table contains all logically plausible combinations of conditions. It has 2k rows, k being the number of causal conditions. Combinations with case membership scores greater than 0.5 are assigned to that combination. Finally, a logical reduction of statements is obtained. During this process, rows are simplified based on two parameters: coverage (i.e. empirical relevance of the solution) and consistency (i.e. the extent to which cases sharing similar conditions have the same results). Both measures range from 0 to 1. Recommended values for acceptable consistency and coverage are 0.75 and 0.45, respectively (Ragin, 2008).
Table 9 contains the three distinct recipes that result from the analysis, verifying the initial hypothesis for the existence of different successful combinations of factors. It is notable that although all three configurations are equally valid, the third (configuration 3) has the highest raw coverage value (0.522) and therefore covers a greater proportion of cases in the sample. The distinction between core and peripheral conditions is used to systematise comparison of the solutions (Fiss, 2011; Ragin, 2008). Core conditions depict the vital causes with robust causal relationships with the outcome of interest, while peripheral conditions are more expendable as they are contingent on specific segments. The solution coverage is 0.661, while the solution consistency is 0.862. All the three configurations also show acceptable consistency scores greater than or equal to 0.828. Raw coverage figures are notably high, implying that the degree to which each recipe explains the outcome is sound. However, unique coverage figures are rather low, suggesting that the proportion of cases that are exclusively explained by the configuration is low. In other words, there are cases in the sample that emerge from a combination of different recipes.
Antecedent Conditions |
Consistency |
Coverage |
OC |
0.699 |
0.690 |
~ OC |
0.493 |
0.530 |
LEAD |
0.761 |
0.801 |
~ LEAD |
0.390 |
0.393 |
OSss |
0.620 |
0.603 |
~ OSss |
0.563 |
0.615 |
OSspc |
0.628 |
0.596 |
~ OSspc |
0.562 |
0.633 |
Antecedent Conditions |
Configurations |
||
1 |
2 |
3 |
|
Organisational capacity |
|
||
Leadership |
|
|
|
Organisational structure-systems & structures |
|
||
Organisational structure-span of control |
|
||
Raw coverage |
0.437 |
0.447 |
0.522 |
Unique coverage |
0.038 |
0.030 |
0.116 |
Consistency |
0.867 |
0.859 |
0.914 |
Solution coverage |
0.661 |
||
Solution consistency |
0.862 |
||
Frequency threshold |
3.000 |
||
Consistency threshold |
0.828 |
Black circles indicate the presence of a condition, and circles with
indicate its absence. Blank spaces indicate “don’t care”. All conditions in the table are core conditions.
Table 9 further shows that leadership is one of the most relevant factors accounting for PMS in DCs. This factor stands as a core condition and appears in all three configurations, although it is not sufficient per se, requiring its combination with the absence of span of control (configuration 1), the absence of systems and structures (configuration 2), or the presence of organisational capacity (configuration 3).
Diving deeper into each of the configurations, we observe that in configuration 1, the presence of leadership combines with the absence of organisational structure (span of control), both being core conditions. This implies that with fewer people, reduced centrality and less administrative paperwork, competent project managers and/or leadership are critical.
The presence of leadership is also determinant in configuration 2 (core condition). In this case, the path suggests the combination of strong leadership with the absence of organisational structure (systems and structures) (also as a core condition). This path includes those projects in which leadership compensates for underdeveloped operational processes within the organisation, with systems and structures being unclearly defined. Strong guidance during the different stages in a project (planning, execution and implementation) can overcome organisations’ poor formalisation, inexplicit rules, regulations, policies and procedures governing organisational activities, improper communication flow and underdeveloped systems.
Finally, configuration 3 embraces those cases in which both leadership and organisational capacity are present. This solution indicates that although strong leadership is a must, it might not be enough, requiring strong organisational capacity. This situation (i.e. the need for organisational capacity) is particularly aggravated when managing complex projects, which typically entail a high level of uncertainty and involve many different stakeholders. In this scenario, the organisation’s ability to, for instance, execute the project effectively, delivering services/products that both satisfy present customer expectations and anticipate future demand, is of utmost importance, even if the organisation scores high for leadership.
4.3 Robustness check
To further explore the main features associated with PMS, similar to Blaskovics (2014), we mimic QCA using structural equation modelling (SEM). We are aware of the existing controversies that this practice entails (Zhao & Yan, 2020), yet we argue that the comparison is meaningful in that it illustrates how QCA—which is based on a fundamentally different approach to causation—can complement traditional analysis performed using SEM approaches. Therefore, the results presented below should be carefully interpreted, as each method is grounded upon a different conceptual perspective.
Firstly, we conducted an analysis of the four factors as dimensions of a second-order confirmatory factor analysis (CFA). The model was estimated using the robust maximum likelihood method from the asymptotic variance–covariance matrix. The fit statistics obtained in the measurement model estimation are shown in Table 10, and the results obtained converged towards the factors established in the validation of the scale. This table also reports the coefficients for the independent relationships established by the model and its t-values. The Satorra–Bentler χ² was 314.87, with 179 degrees of freedom and a p-value of 0.000; χ²/df was 1.76, which was below the acceptable limit of 5. The root mean square error of approximation (RMSEA) was 0.060; the comparative fit index (CFI) was 0.952; while the 90 per cent confidence interval of RMSEA was reported to be between 0.048 and 0.070. Taking the significance of the robust χ² statistic with caution and bearing in mind the global indicators, we concluded that the global fit was acceptable.
Table 10: Confirmatory factor analysis
Dimension |
Item |
Load |
t-value |
r2 |
Organisational capacity |
OC1 |
0.785 |
– |
0.617 |
OC2 |
0.714 |
12.890 |
0.509 |
|
OC3 |
0.672 |
9.570 |
0.451 |
|
OC4 |
0.764 |
11.570 |
0.584 |
|
Leadership |
LEAD1 |
0.661 |
– |
0.437 |
LEAD2 |
0.877 |
11.590 |
0.769 |
|
LEAD3 |
0.798 |
10.370 |
0.637 |
|
LEAD4 |
0.782 |
9.520 |
0.611 |
|
Organisational structure-systems & structures |
OSss1 |
0.768 |
– |
0.589 |
OSss2 |
0.641 |
8.800 |
0.411 |
|
OSss3 |
0.748 |
11.560 |
0.560 |
|
OC5 |
0.764 |
8.270 |
0.584 |
|
Organisational structure-span of control |
OSspc4 |
0.673 |
– |
0.452 |
OSspc5 |
0.660 |
6.650 |
0.436 |
|
OSspc6 |
0.722 |
8.060 |
0.521 |
|
Standardised Coefficient |
t-value |
r2 |
||
Project management success |
Organisational capacity |
0.653 |
10.380* |
0.822 |
Leadership |
0.789 |
9.330* |
||
Organisational structure-systems & structures |
0.138 |
1.319 |
||
Organisational structure-span of control |
-0.217 |
-2.050* |
||
Goodness of fit summary |
||||
Satorra–Bentler scaled X2 |
314.868 |
|||
Degrees of freedom (df) |
179 |
|||
p-value |
0.000 |
|||
X2/df |
1.759 |
|||
Comparative fit index (CFI) |
0.952 |
|||
Root mean-square error of approximation (RMSEA) |
0.060 |
|||
90% confidence interval of RMSEA |
(0.048 – 0.070) |
When using SEM to regress the factors against the outcome of interest (PMS), the results revealed that organisational capacity has a significant causal relationship with PMS (β = 0.653, t-value = 10.380), meaning that project-oriented organisations with improved capacity are likely to experience a higher PMS rate, and vice versa. Similarly, leadership is found to be a significant predictor of PMS (β = 0.789, t-value = 9.330). This positive relationship suggests that the type, competence and skills of leaders of project-oriented organisations in DCs are critical; therefore, training in leadership skills must be guaranteed in order to ensure PMS. This result is consistent with the QCA, in which leadership was found to be a core condition, present in all configurations.
The SEM model also showed that organisational structure (systems and structures) positively influences PMS, but this relationship is not statistically significant (β = 0.138, t-value = 1.319). In contrast, organisational structure (span of control) is found to have a negative impact on PMS (β = -0.217, t-value = -2.050), which means that the likelihood of it leading to PMS is higher in project-oriented organisations that are more flexible (less bureaucratised and less administrative paperwork), decentralised and organised in small project teams. This result is consistent with the findings from the QCA, where in addition to strong leadership, an organisational structure characterised by small teams, reduced centrality and fewer administrative barriers is preferred.
Concluding
6. Contribution and concluding remarks
Evidence shows that PM in many DCs tends to experience high levels of failure. Aiming to offer practical insights for PM in these countries to help them leverage their limited resources, this study examines how different combinations of major factors signalled in the PM literature (i.e. organisational capacity, organisational structure and leadership) are characterised in DCs and how they should be combined in order to yield PMS. The results provide intriguing policy recommendations for project initiators in DCs.
One of the key findings of this study is the role played by organisational capacity in shaping PMS. When examining the net effect in the SEM model, we observed that this factor positively influences PMS. That is, project-oriented organisations that have the capacity to fulfil their mission through a blend of sound management, strong governance, effective utilisation of skills, assets and resources, and a persistent rededication to assessing and achieving results are more likely to achieve PMS. The direction of this effect is aligned with the findings of Cox et al. (2018) and Rankonyana (2015), where the capacity to communicate effectively and transfer knowledge was directly linked to the quality delivered, the time spent and, ultimately, the overall management of a project. At this point, it is worth highlighting that our results from the QCA open new avenues for project-oriented organisations that have limited organisational capability and, instead of preventing them from achieving PMS, we observe that their route to PMS is tied to strong leadership.
Consequently, before accepting any project contract in DCs, project-oriented organisations need to assess the project thoroughly and determine whether their capacity suffices. This capacity includes competent human resources (which, in turn, are linked with leadership), IT infrastructure, as well as networks and linkages with other organisations and groups. Indeed, a major limitation in implementing and managing projects in DCs upon completion is not financial resources but the capacity of the project-oriented organisation (Bjorvatn & Wald, 2018). It is therefore recommended that organisations clearly define their procedures and internal systems, as excessive rigidity and ambiguity might come at the expense of flexibility and thus constrain the capacity of the organisation to respond rapidly to the specificities of PM in DCs.
Another relevant outcome of this study is that the interpretation of the role played by organisational structure is not straightforward. Our results suggest that the absence of span of control might not necessarily be a limitation; on the contrary, in the presence of strong leadership, shortcomings associated with less systematised procedures and more radial and scattered management systems might not be an impediment to achieve PMS: instead, they offer the flexibility that DCs’ PM requires. Likewise, well laid down procedures, rules, regulations, policies, and established communication channels are not a must in DCs.
In fact, projects in DCs seems to benefit when each project is conceived as a unique endeavour and, instead of following a rigid structure to monitor it, each project is individually designed and tracked using the instruments and techniques that are most suitable to complete it successfully. Yet, for this flexibility to work, again, strong leadership is needed. This means that reducing bureaucracy and dismantling fixed structures is preferred, which leads us to advocate the use of decentralised and loose structures in which employees work in small teams. The adoption of electronic systems can help in this direction since these systems reduce bureaucracy and promote monitoring and transparency.
Finally, we observed that leadership is the backbone of PMS. The configurations obtained in the QCA suggest that if a project-oriented organisation is bureaucratic and is in a situation where a project manager supervises too many subordinates, it cannot successfully manage a project in a DC unless it has leadership with the required competences. Likewise, the presence of organisational capacity alone is not enough, requiring a strong figure leading the project during its conception, planning, execution and implementation (Larson & Gray, 2014). The findings from the SEM analysis reinforce that the leadership style, behaviour and competence exhibited by leaders are paramount to success, especially in a geographical area where the structures, processes and frameworks of the project-oriented organisations are not well laid down.
5.1 Implications
From a managerial perspective, the findings from this study indicate that, as a matter of policy, project-oriented organisations in DCs should rely on qualified project managers to lead projects. Managers who lead project teams in DCs need to have sound knowledge in the field and a deep understanding of the geographical context in which the project is being managed. The leaders should possess both technical and soft skills/competencies. Arguably, not all project managers might possess these skills as PM courses tend to be more oriented towards the specificities and technicalities of the job. However, soft skills should not be overlooked, it being imperative to offer adequate training for continuous professional development in these abilities, which will help project managers to lead effectively (Ahadzie et al., 2009).
Furthermore, leaders of project-oriented organisations conducting projects in DCs should have the ability to integrate team members around the organisational goals, define roles and responsibilities, communicate with key stakeholders, and motivate and inspire the team (Siles, 2021). They should provide the required framework and structures that allow projects to be viewed as an integrated system of activities that are interrelated and that develop and evolve over the entire project lifecycle.
From the findings of this study, the causal relationship for organisational structure with the outcome was found not to be straightforward. Specifically, while there is support for the effect of organisational structure (span of control) on PMS, when examining organisational structure (systems and process) the relationship is not significant. This implies that the theory underlining Hyväri’s (2006) model for PMS can be used in the context of DCs, though with some adjustments. The need for this fine-tuning is obviously a result of the differences in context in the application of the model.
Finally, from the findings of this study, one can observe the existence of distinct pathways, suggesting that there is no unique formula, but that different situations might require the adoption of diverse PM strategies to manage projects in DCs successfully.
6. Contribution and concluding remarks
The evidence of multiple construction projects failing or being abandoned without finishing calls for a better understanding of the challenges PM face in DCs. With the ultimate purpose of making recommendations for policy and practice to overcome these problems, the aim of this study has been to validate the relevance and effect in PMS in DCs of three major factors identified in the literature—organisational capacity, organisational structure and leadership. To do so, we have revised the extant literature and particularised the abovementioned factors in the peculiar and complex setting of DCs. Our findings reveal that organisational capacity, organisational structure and leadership might combine in different ways, it being possible to observe a set of equally valid configurations that project managers should consider in order to manage projects in DCs successfully.
The original contribution of this research stems from putting a spotlight on how projects can be successfully managed in DCs. By highlighting which factors are critical and their impact on PMS, it is expected that the problem of high-level PM failures in DCs can be better addressed. Unsuccessful project delivery is a reflection of flawed management practices. We expect that deepening knowledge on the major factors that affect PMS in DCs will help both policymakers and managers of project-oriented organisations in these countries to develop more appropriate policies and use resources more efficiently.
Methodologically, this study contributes to the PM literature by using novel alternative analytical techniques to create an appropriate model that catalyses the adoption of appropriate approaches that support the efficient management of projects in DCs. The study makes a theoretical contribution by providing new insights to add to the existing literature in the specific context of PM in DCs. In this regard, this study is one of the very first to provide a model that ca be used by project-oriented organisations to achieve PMS in DCs.
6.1 Limitations and suggestions for future research
Although this research was conducted using a rigorous process, the study is not free of limitations, which, in turn, present new opportunities for future research. For instance, despite being able to characterise organisational capacity, organisational structure and leadership in DCs, our analyses did not consider other factors, such as culture, politics and the project’s external environment, that might play a role in shaping PMS. Future studies might consider expanding the model and including these types of contextual factor. Another limitation is that since the instrument used to capture respondents’ perceptions regarding the components of the factors influencing PMS in DCs is quantitative, a degree of subjectivity might be contained in the data collected. Future works might consider triangulating the data with interviews with relevant stakeholders, including both experienced researchers in PM in DCs and major players (i.e. practitioners) from these countries. Last but not least, our dataset is cross-sectional. New research endeavours might consider analysing the effect of specific interventions (e.g. training in leadership skills) in the attempt to improve PMS.
Acknowledgments
References
References
Ackah, D. (2020). Roots of Project Failure and Abandonment in Developing Countries. Project Management Scientific Journal, 4(4), 45-61.
Ahadzie, D. K., Proverbs, D. G., Olomolaiye, P. O., & Ankrah, N. A. (2009). Competencies required by project managers for housing construction in Ghana: Implications for CPD agenda. Engineering, Construction and Architectural Management, 16(4), 353-375. https://doi.org/10.1108/09699980910970842
Ahmed, R., & Mohamad N. A. (2016). Exploring the relationship between multi-dimensional top management support and project success: An international study. Engineering Management Journal, 28(1), 54-67. https://doi.org/10.1080/10429247.2015.1136525
Alias, Z., Zawawi, E. M., Yusof, K., & Aris, N. M. (2014). Determining critical success factors of project management practice: A conceptual framework. Procedia - Social and Behavioral Sciences, 153, 61-69. https://doi.org/10.1016/j.sbspro.2014.10.041.
Alrik, T & Dușa, A. (2013). Qualitative comparative analysis with R. A user’s guide. New York; Heidelberg; Dordrecht; London: Springer.
Amoah, A., & Marimon, F. (2021). Project managers as knowledge workers: competencies for effective project management in developing countries. Administrative Sciences, 11(4), 131. https://doi.org/10.3390/admsci11040131
Amoah, A., Berbegal-Mirabent, J., & Marimon, F. (2021). Making the management of a project successful: Case of construction projects in developing countries. Journal of Construction Engineering and Management, 147(12), 04021166. https://doi.org/10.1061/(ASCE)CO.1943-7862.0002196
Aniagyei, W. (2011). The effect of organizational structure on project performance: the case of Aga Ltd. A Doctoral dissertation, Kwame Nkrumah University of Science and Technology, Ghana.
Asiedu, R. O., & Adaku, E. (2019). Cost overruns of public sector construction projects: a developing country perspective. International Journal of Managing Projects in Business, 13(1), 66-84. https://doi.org/10.1108/IJMPB-09-2018-0177
Bjorvatn, T., & Wald, A. (2018). Project complexity and team-level absorptive capacity as drivers of project management performance. International Journal of Project Management, 36(6), 876-888. https://doi.org/10.1016/j.ijproman.2018.05.003
Blaskovics, B. (2014). Aspects of projects success: The case of a multinational company. PMUni Conference Vienna, 14 May.
Budget of the Republic of Ghana. (2019). Budget highlights: A stronger economy for jobs and prosperity. Accessed March 18, 2022. https://www.pwc.com/gh/en/assets/pdf/2019-budget-highlights.pdf
Burke, C. S., Stagl, K. C., Klein, C., Goodwin, G. F., Salas, E., & Halpin, S. M. (2006). What type of leadership behaviors are functional in teams? A meta-analysis. The Leadership Quarterly, 17(3), 288-307. https://doi.org/10.1016/j.leaqua.2006.02.007
Choi, Y. J. (2009). Application of fuzzy-set theory in social sciences. Journal of Governmental Studies, 15(3), 307-336.
Connolly, P., & Lukas, C. (2002). Strengthening non-profit performance: A funder's guide to capacity building. St. Paul, MN: Amherst H. Wilder Foundation.
Cox, K., Jolly, S., Van Der Staaij, S., & Van Stolk, C. (2018). Understanding the drivers of organizational capacity. Cambridge, UK: Rand Corporation.
Damoah, I. S., and Akwei, C., (2017). Government project failure in Ghana: a multidimensional approach. International Journal of Managing Projects in Business, 10(1), 32-59. https://doi.org/10.1108/IJMPB-02-2016-0017
Damoah, I. S., and D. K. Kumi. (2018). Causes of government construction projects failure in an emerging economy: Evidence from Ghana. International Journal of Managing Projects in Business, 11 (3), 558-582. https://doi.org/10.1108/IJMPB-04-2017-0042
Darko, E., & Löwe A. (2016). Ghana’s construction sector and youth employment. London: Overseas Development Institute.
dos Santos, P. M., & Cirillo, M. Â. (2021). Construction of the average variance extracted index for construct validation in structural equation models with adaptive regressions. Communications in Statistics-Simulation and Computation, 50(12), 1-13. https://doi.org/10.1080/03610918.2021.1888122
Dulewicz, V., & Higgs, M. J. (2004). A new instrument to assess leadership dimensions and styles. Selection & Development Review, 20(2), 7-12. http://eprints.soton.ac.uk/id/eprint/51494
Ekundayo, D., Jewell, C., & Awodele, O. A. (2013). Executive project management structure and the challenges facing its adoption in the Nigerian construction. International Journal of Architecture, Engineering and Construction, 2(3), 158-169. http://dx.doi.org/10.7492/IJAEC.2013.015
Engle, N. L. (2011). Adaptive capacity and its assessment. Global Environmental Change, 21(2), 647-656. https://doi.org/10.1016/j.gloenvcha.2011.01.019
Essilfie-Baiden, E. (2019). Challenges of project management in developing countries. Project Management Scientific Journal, 3(6), 84-88.
Fiss, P. C. (2011). Building better causal theories: A fuzzy set approach to typologies in organization research. Academy of Management Journal, 54(2), 393-420. https://doi.org/10.5465/amj.2011.60263120
Fong, N. K., Wong, L. Y., & Wong, L. T. (2006). Fire services installation related contributors of construction delays. Building and Environment, 41, 211-222. https://doi.org/10.1016/j.buildenv.2005.01.004
Fornell, C. & Larcker, D. F. (1981). Evaluating structural equation models with unobservable variables and measurement error. Journal of Marketing Research, 18(February), 39-50. https://doi.org/10.1177/002224378101800104
Fortune, J., & White, D. (2006). Framing of project critical success factors by a systems model. International Journal of Project Management, 24, 53-65. https://doi.org/10.1016/j.ijproman.2005.07.004.
Frefer, A. A., Mahmoud, M., Haleema, H., & Almamlook, R. (2018). Overview success criteria and critical success factors in project management. Industrial Engineering Management, 7(10), 244. https://doi.org/10.4172/2169-0316.1000244
Hair, J. F., Black, W. C., Babin, B. J. & Anderson, R. E. (2010). Multivariate data analysis (7th ed.), New York: Pearson.
Hanisch, B., Lindner, F., Mueller, A., & Wald, A. (2009). Knowledge management in project environments. Journal of Knowledge Management, 13(4), 148-160. https://doi.org/10.1108/13673270910971897
Hyväri, I. (2006). Project management effectiveness in project-oriented business organizations. International Journal of Project Management, 24, 216-225. https://doi.org/10.1016/j.ijproman.2005.09.001
Ika, L. A. (2012). Project management for development in Africa: Why projects are failing and what can be done about it. Project Management Journal, 43(4), 27-41. https://doi.org/10.1002/pmj.21281
International Project Management Association (2006). IPMA competence baseline, version 3.0. International Project Management Association, Nijkerk.
Inuwa, I. I., & Kunya, S. U. (2015). Project management theory exploration for indigenous contractors’ project planning in Nigeria. International Journal of Applied Science and Technology, 5(3), 12-19.
Islam, M. A., Jantan, A. H., & Saimoon, A. (2017). Factors affecting females’ participation in leadership positions in RMG industry, Bangladesh. International Journal of Scientific and Research Publications, 7(10), 535-542.
Kaiser, H. F. (1960). The application of electronic computers to factor analysis. Educational and Psychological Measurement, 20(1), 141-151. https://doi.org/10.1177/001316446002000116
Kamaruddeen, A. M., Sung, C. F., & Wahi, W. (2020). A study on factors causing cost overrun of construction projects in Sarawak, Malaysia. Civil Engineering and Architecture, 8(3), 191-199. https://doi.org/10.13189/cea.2020.080301
Kerzner, H. R. (2013). Project management: A systems approach to planning, scheduling, and controlling. New York: John Wiley.
Kissi, E., Ahadzie D. K., & Badu E. (2015). Strategies for improving professional project management practices in the Ghanaian construction industry. NICMAR-Journal of Construction. Management, 30(2), 5-9.
Ladhari, R. (2012). The lodging quality index: An independent assessment of validity and dimensions. International Journal of Contemporary Hospitality Management, 24(4), 628-652. https://doi.org/10.1108/09596111211217914
Larson, E. W., & Gray, C. F. (2014). Project management: The managerial process, (6th Ed.). New York: McGraw-Hill Education.
Lee, S. S. (2008). A critique of the fuzzy set methods in comparative social policy (No. 53). COMPASSS Working Paper.
Lussier, R. N., & Achua, C. F. (2013). Leadership: Theory, application, and skill development, (4th Ed.). South-Western, OH: Mason.
Malhotra, N. K. (1999). Marketing research: An applied orientation. London: Prentice-Hall International.
Meredith, J. R., & Mantel Jr, S. J. (2011). Project Management: A Managerial Approach. New York: Wiley Global Education.
Meyer, A. D., Tsui, A. S. & Hinings, C. R. (1993). Configurational approaches to organizational analyses. Academy of Management Journal, 36(6), 1175-1195. https://doi.org/10.5465/256809
Meyer, S. M., & Collier, D. A. (2001). An empirical test of the causal relationships in the Baldrige health care pilot criteria. Journal of Operations Management, 19(4), 403-425. https://doi.org/10.1016/S0272-6963(01)00053-5
Mikkelsen, Y. (2019). Organising the literature in a systematic literature review using factor analysis. Value in Health, 22, S769-S770.
Milosevic, D., & Patanakul, P. (2005). Standardized project management may increase development projects success. International Journal of Project Management, 23(3), 181-192. https://doi.org/10.1016/j.ijproman.2004.11.002
Mukalula, P. M. (1996). Structural adjustment for a competitive environment: Survival strategies for medium sized organization firms in Zambia. International Symposium for the Organization and Management of Organization, Spon, London.
Murch, R. (2001). Project management. Upper Saddle River, New Jersey: Prentice Hall, Inc.
Muriithi, S. M., Waithira, V., & Wachira, M. (2016). Family business founders’ influence on future survival of family businesses. International Journal of Economics, Commerce and Management, 4(1), 560-575. http://repository.daystar.ac.ke/xmlui/handle/123456789/3599
Ochieng, V. O. (2016). Influence of organizational structure on project performance: A case of Taylor Nelson Sofres. A Doctoral dissertation, University of Nairobi, Nairobi, Kenya.
Ofori, D. F. (2013). Project management practices and critical success factors: A developing country perspective. International Journal of Business and Management, 8(21), 14-31. http://dx.doi.org/10.5539/ijbm.v8n21p14
Ofori-Kuragu, J. K., Owusu-Manu, D., & Ayarkwa, J. (2016). The case for the construction industry council, Ghana. Journal of Construction in Developing Countries, 21(212), 131-149. https://dx.doi.org/10.21315/jcdc2016.21.2.7
Owusu-Ansah, M., & Louw L. (2019). The influence of national culture on organizational culture of multinational companies. Cogent Social Science, 5(1), 162-364. https://doi.org/10.1080/23311886.2019.1623648.
Pallant, J. (2010). SPSS Survival Manual: A step by step guide to data analysis using the SPSS program (4th ed.), New York: McGraw Hill.
Pandya, K. D. (2014). The key competencies of project leader beyond the essential technical capabilities. IUP Journal of Knowledge Management, 12(4), 39-48.
Pavlou, P.A., Liang, H., & Xue, Y. (2007). Understanding and mitigating uncertainty in online exchange relationships: A principal-agent perspective. MIS Quarterly, 31(1), 105-136.
Pennings, J. M. (2002). Structural contingency theory: A reappraisal. In S. Clegg (ed.), Central Currents in Organization Theory: Fundamentals and Applications, 3, London: Sage Publications
Podsakoff, P.M., MacKenzie, S.B., Lee, J.-Y., & Podsakoff, N.P. (2003). Common method biases in behavioural research: A critical review of the literature and recommended remedies. Journal of Applied Psychology, 88(5), 879-903.
Project Management Institute (2017). A guide to the project management book of knowledge (PMBOK), (6th Ed.). Newtown Square: Project Management Institute Inc.
Radujković, M., & Sjekavica, M. (2017). Project management success factors. Procedia Engineering, 196, 607-615. https://doi.org/10.1016/j.proeng.2017.08.048
Ragin, C. C. (2008). Redesigning social inquiry: Fuzzy sets and beyond. Chicago: University of Chicago Press.
Rankonyana, L. (2015). An analysis of the effect of organizational capacity on organizational performance in project implementation: A case of the Organization of Rural Associations for Progress (ORAP). A Doctoral dissertation, Stellenbosch University, Stellenbosch.
Robbins, S. P., & Barnwell, N. (2006). Organization theory: Concepts and cases. Australia: Pearson Education.
Sablynski, C. J. (2012). Foundation of organizational structure. New Yolk: Sage.
Salam, M. A., Ali, M. & Kan, K. A. S. (2017). Analyzing supply chain uncertainty to deliver sustainable operational performance: Symmetrical and asymmetrical modeling approaches. Sustainability, 9(12), 2217. https://doi.org/10.3390/su9122217
Schneider, C. Q. & Wagemann, C. (2012). Set-theoretic methods for the social sciences: A guide to qualitative comparative analysis. Cambridge: Cambridge University Press.
Sekaran, U. (2009). Research methods for business (5th Ed). Hoboken, New Jersey: John Wiley & Sons.
Shemwell, J. T., Chase, C. C., & Schwartz, D. L. (2015). Seeking the general explanation: A test of inductive activities for learning and transfer. Journal of Research in Science Teaching, 52(1), 58-83. https://doi.org/10.1002/tea.21185
Sienkiewicz-Malyjurek, K. (2016). Determinants and attributes of leadership in the public safety management system. Procedia Economics and Finance, 39, 115-121. https://doi.org/10.1016/S2212-5671(16)30254-4
Siles R. (2021). Definition of project success: Project management for development. Retrieved on March 23rd, 2022 at https://www.pm4dev.com/pm4dev-blog/blogger/rodolfosiles.html
Tesfaye, E., Lemma, T., Berhan, E., & Beshah, B. (2017). Key project planning processes affecting project success. International Journal for Quality Research, 11(1), 159-172. https://doi.org/10.18421/IJQR11.01-10
Turner, J. R. (2006). Towards a theory of project management: The nature of the project governance and project management. International Journal of project management, 2(24), 93-95. https://doi.org/10.1016/j.ijproman.2005.11.008
Ubani, E. C. (2012). Evaluating the effects of organizational structure on the effective delivery of civil engineering projects. Interdisciplinary Journal of Contemporary Research in Business, 4(6), 1284-1296.
Ullah, K., Abdullah, A. H., Nagapan, S., Sohu, S., & Khan, M. S. (2018). Measures to mitigate causative factors of budget overrun in Malaysian building projects. International Journal of Integrated Engineering, 10(9), 66-71. https://doi.org/10.30880/ijie.2018.10.09.032
Walker, D. H., & Shen, Y. J. (2002). Project understanding, planning, flexibility of management action and construction time performance: two Australian case studies. Construction Management & Economics, 20(1), 31-44. https://doi.org/10.1080/01446190110089691
Wallace, S., (2007). Project structure and organisation. Accessed on July 12, 2020 at https://www.epmbook.com/structure.htm
Williams, T. (2017). The nature of risk in complex projects. Project Management Journal, 48(4), 55-66. https://doi.org/10.1177/875697281704800405
Woodside, A. G. (2010). Bridging the chasm between survey and case study research: Re- search methods for achieving generalization, accuracy, and complexity. Industrial Marketing Management, 39(1), 64–75. https://doi.org/10.1016/j.indmarman.2009.03.017
World Bank (2019). Annual World Development Report. Accessed on December 7, 2020 at https://www.worldbank.org/en/publication/wdr2019
Yanwen, W. (2012). The study on complex project management in developing countries. Procedia, 25, 1547-552. https://doi.org/10.1016/j.phpro.2012.03.274
Zhao, J., & Yan, C. (2020). User Acceptance of Information Feed Advertising: A Hybrid Method Based on SEM and QCA. Future Internet, 12(12), 209. https://doi.org/10.3390/fi12120209.